Understanding the future through the land around us
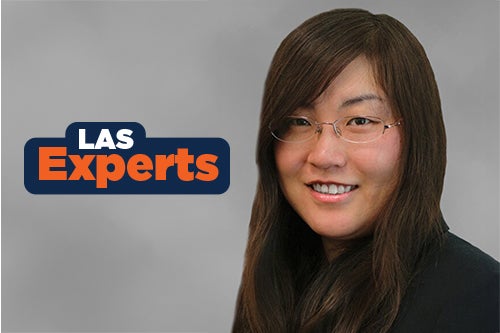
On any given drive across the countryside you may see cornfields or a forest. Chunyuan Diao, a professor in the Department of Geography & Geographic Information Science, sees a complex terrestrial ecosystem that can provide clues to improving food security, climate change, and sustainability.
Diao joined the U of I in 2017 and she has become a highly respected reseacher of ground dynamics. She received the National Science Foundation CAREER award and earlier this year was named a Fellow of the American Association of Geographers (AAG). She has developed a reference data framework, CropSight, to help us better understand the land around us.
What are you working on now? Why are you passionate about this area of study?
My research mainly lies at the confluence of remote sensing, GIScience, and biogeography. My current research focuses on computational remote sensing of terrestrial ecosystem dynamics at local to global spatial scales, and daily to decadal temporal scales. I am particularly passionate about advancing computational remote sensing paradigms in characterizing land surface patterns and processes, underlying mechanisms, and subsequent feedbacks to the atmosphere. My work combines remote sensing, process-based models, field observations, artificial intelligence, high-performance and cloud computing, to study ecosystem structures, functions, and responses to climate change and human activities. My ongoing research traverses varying types of ecosystems, including natural (e.g., forest), human-dominated (e.g., agriculture), and disturbed (e.g., species invasion) ecosystems. Current research foci include computational remote sensing, multi-scale land surface phenology, intelligent agriculture, and invasive species and biodiversity.
What challenges have you overcome to better understand this area of research?
One significant challenge in geography and remote sensing research is the difficulty in acquiring ground truth reference data of land covers/land uses at large scales. In agricultural remote sensing, the difficulty in collecting accurate ground truth data of crop types over space and time has hindered many agricultural studies, and the lack of crop type labels over wide geographical regions remains a major obstacle. To overcome this challenge, we innovatively leveraged the street view imagery, and developed a novel framework, called CropSight, to retrieve the object-based crop type ground truth. CropSight is a unique national-scale crop ground reference data repository and embodies a wealth of season-long remotely sensed crop growth and environmental attributes across crop growing locations for most crop types in the U.S. CropSight can be generalized to continental and global scales, and will be used as a large-scale, systematic, and consistent ground reference data repository. Addressing this challenge opens up new opportunities to many agricultural remote sensing studies, including crop type mapping, condition monitoring, and yield estimation.
Please describe a moment when your professional career changed direction.
Since I joined our university, I have largely expanded my research area to agriculture, an exciting area of our campus research strength with significant societal impacts. The stronger emphasis of my research on agricultural remote sensing is owing to the significance and its impacts on food security. The global demand for agricultural crops is rapidly increasing with the continuing growth of the worldwide population, posing significant threats to food security. The escalating crop demand consequently results in the intensification and extensification of agricultural productions, which overstrains ecosystem services and causes drastic environmental degradation. So there is an increasing need to transform agricultural systems into resource-efficient systems that are both productive and environmentally sustainable.
I am excited to dive into this new research area. Built upon the concepts of digital agriculture and precision agriculture, I am particularly interested in advancing monitoring and modeling constituents underlying intelligent agricultural systems, with the synthesis of multi-source remote sensing, crop and ecosystem models, deep learning, and farm experiments. My research has focused on intelligent field-level crop type mapping, crop phenology and condition retrieval, crop yield estimation and forecasting, and farming practices prioritization from local to global scales. I also investigate the influence of changes in climate and extreme weather on agroecosystems to advance the science underpinning resilient and sustainable agriculture.